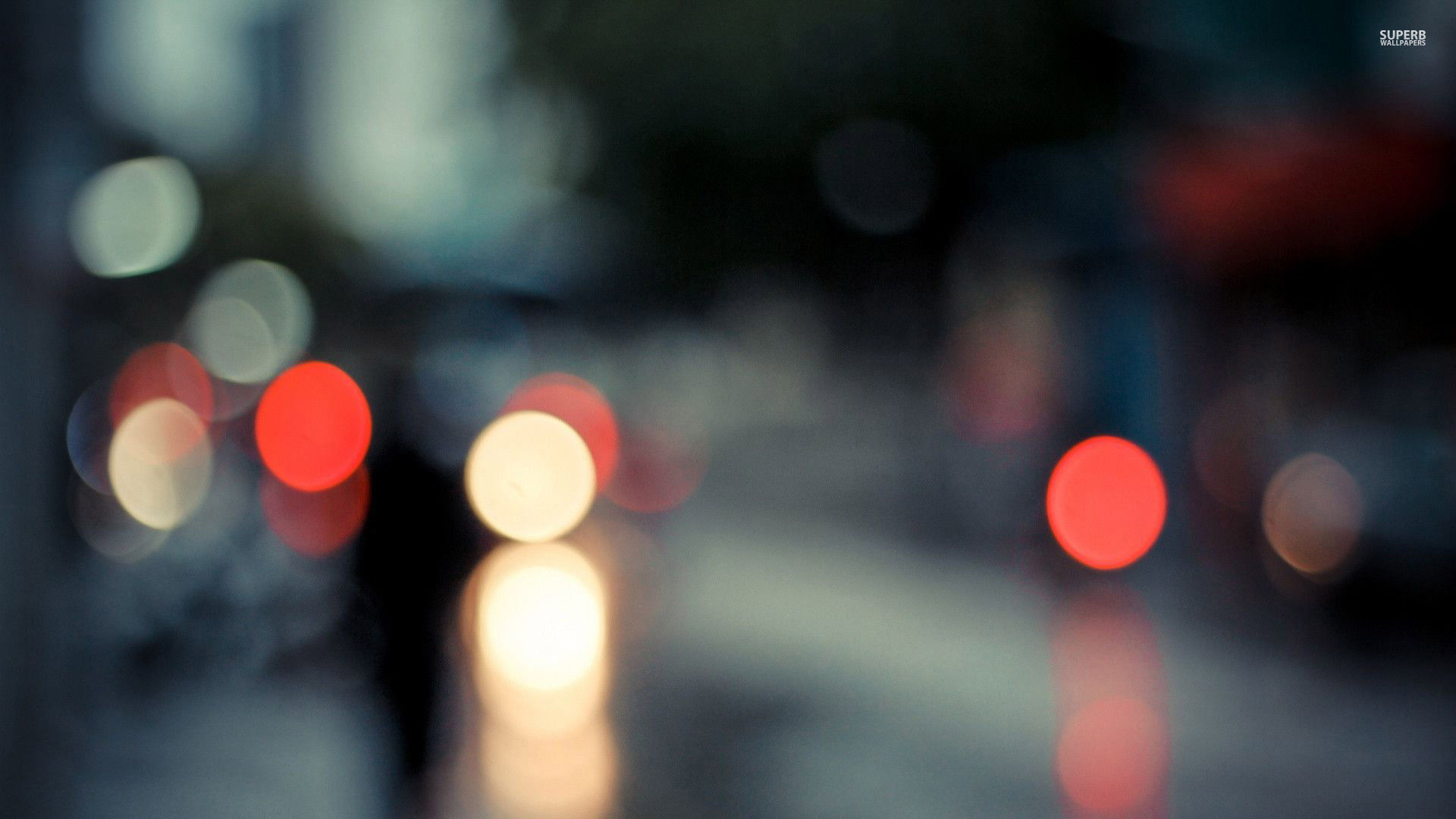
Overconfidence
Study: Mamassian, P. (2008). Overconfidence in an Objective Anticipatory Motor Task.
Introduction:
Overconfidence is a widespread topic in studies since it can have dramatic consequences in several fields. We can think of driving (feeling that one is capable of driving and texting at the same time for example), stock exchange trading (waiting to sell stocks on the market because one is convicted that the rate is going to raise) and so on.
The main question for researchers is whether it can be reliable to collect information on overconfidence based on self-report evaluation or if it exists a way to collect data based on an objective measure. Also, the idea was to correlate the overconfidence with the extent of their uncertainty.
Context:
So far, evaluating overconfidence has been essentially made through self-report results. Obviously, this method can trigger biases in the analysis since people’s overconfidence reports is based on their own overconfidence behavior. Then, the originality of this study comes from the method that has been implemented in order to report an objective measure of overconfidence. This was enabled thanks to the set up of the simple motor task evaluation.
Moreover, this aim of going from a subjective to an objective measure is consistent with the current paradigm which consists in thinking that overconfidence is involved in the human decision-making process and not only limited to subjective ratings in cognitive tasks.
In order to set up the study, the definition of overconfidence used is the following: “overconfidence refers to the human tendency to overestimate one’s own abilities and knowledge” (M. Alpert & H. Raiffa, 1982).
Method:
Ten participants from 23 to 54 years old (M = 32) took part in the experiment.
Four screen were displayed one after the other (with a constant stimulus onset asynchrony of 500 ms) and on the last one, 6 dots represent a hexagon. The task was to anticipate the apparition of the last screen with the hexagon by hitting a key in a right lapse of time (+ or – 0.25 seconds).
A reward of 100 points was given if the anticipation was good (i.e. Included in a narrow temporal interval) and a loss of 200 points otherwise.
Experimenters implemented three conditions: no-penalty, late-penalty and early-penalty.
The model used to describe participants’ behavior is the basic convolution of two functions: expected gain = hitting uncertainty * utility function. g(t) = h(t) * u(t). The hitting variability follows a Gaussian distribution. This prediction comes from a model where the SD of the motor uncertainty is 25 ms.
Results:
First of all and with respect to the results, we can point out that participants overestimated the interval of time between the events, which generated a time order error. Indeed “the perception of time is a crucial factor when individuals have to make decisions and consider the outcomes associated with their choices” (M. Wittman & M. Paulus, 2007). In their study, Wittman & Paulus (2007) link the overestimation of time with the impulsivity of subjects. They account for the fact that the emotional distress of subjects leads them to allocate more attentional resources to time, which distort how they perceive time, hence the overestimation. Let us now return to our study.
Authors have explained the overestimation of the interval between the first two events by a recalibration of the visuo-motor system, but we can now wonder if the impulsivity of subjects can have a role to play here. This is why it could be interesting to reiterate this experiment including an impulsivity measuring-scale.
Moreover, another important finding is about the participants hitting time variability. In fact, the hitting time variability decreased over time, which conducted to an increase of expected scores. For all that, participants’ scores remained significantly below the expected scores; thereby authors argued that subjects did not get wind of how to stave off the penalty periods. However, authors based all the results on the reliability of the model they used; they assert that once they were aware of the hitting variability of a participant in a given session, they could evaluate its expected optimal behavior.
What if the model used in this study was not sure enough? Indeed, in this model, authors took into account the utility function and the hitting variability of participants in order to obtain their expected optimal behavior. On the other hand, they did not consider other factors relative to the participants’ emotional state or their potential impulsivity, for instance.
Actually, it has been proved that our emotional state alters the way we make decisions. In 1999, Bechara et al. demonstrated that the apparition of biases in decision-making was due to the role played by the amygdala. In 2000, they combined these results with those found by Cahill et al. in 1995 showing that the amygdala was also important to make emotions improving memory. Thus, they proposed that: “in the amygdala, the mechanisms through which emotion modulates memory and decision making may be inseparable” (Bechara et al. 2000). This proposition is consistent with previous theories proving the primary role for emotion in decision-making (Zajonc, 1984).
Besides, in order to get the reason why participants’ performance was suboptimal, authors led two further experiments. The first experiment tested the potential underestimation of the own motor variability of participants, and the second experiment tested the potential underestimation of the lost of the penalty periods. Results have shown that participants did take into account the potential lost due to responses made too late or too early, but they were not able to manage to get optimal hitting times. As we proposed before, this can be due to the low reliability of the model used, but if we consider this model as reliable, this prove that participants don’t know their own motor variability.
Thus, another important fact to point out is about the potential learning effect on participants – which means that participants should be more experimented and fast by executing the same task in a repeated way -, which could explain the increase of their performance. Authors concluded that it may not be possible to learn how to hit at the right time.
For this point, we both have our own point of view. On the one hand, Celia’s point of view:
In my opinion, authors’ point of view concerning the absence of learning effect here is coherent with their findings. Indeed, even though participants’ variability increased over time, results were not as high as expected with respect to the model used. In this way, if participants learned the behavior they had to have (i.e hit at the right time), their performances would have been almost perfect at the end of the experiment. This is why here, it is better to talk about an effect of sessions, which permits, to participants to improve their responses.
Otherwise, one of the two further experiments led by authors conducted to the fact that participants’ own motor variability was implicit. Hence, it is impossible to learn an implicit behavior.
On the other hand, Gregoire’s point of view:
I don’t understand why authors end up with the conclusion that participants manage to do better in the further experiments arguing at the same time that there was no learning effect. Indeed, according to the authors, they adapted their behavior but did not to reach an optimal response. Then, as far as I am concerned, I would say that there exists a true learning effect for two reasons:
-
A feedback was provided each time participants hit the key too early or too late. The purpose of the feedback should be to create a learning effect.
-
Participants improved their score by adapting themselves because they acquired knowledge from the first task. Even if they did not reach an optimal response, they improved their score. Authors mention the sub optimality of responses. This statement can lead to another debate.
Mathematical problem:
Authors concluded that participants responded in a suboptimal way. But to me, they responded in a sub maximum way. The difference lies in the fact that, to me, participants actually responded optimally because they improved, but did not reach the expected best response because their constraint was their cognitive motor adaptation.
At a mathematical level, an optimum is reach according to a constraint (usually a budget constraint in microeconomics), but a maximum is deprived of constraint. Within a graph, an optimum is reached before a maximum, specifically because the optimum point is bounded by its constraints, belonging to the reference universe. Therefore, the expected best-response that was computed thanks to the mathematical model used in the study (g(t) = h(t) * u(t)) embodies the maximum in the function. Whereas thanks to their improvement, participants reached an optimal point bounded by their cognitive and motor constraint at this time of the experiment. Indeed, we can think that they would have even done better if they repeated again the experiment. This is where the learning effect intervenes.
Criticism:
First, regarding the experiment that has been conducted, we are not informed whether the points earned at the end were converted into money or not. We can think positively but still we are not aware of the conversion rate. If we assume that the endowment of 5 000 points is purely fictitious and won’t be converted into money (after fluctuation, depending on the results in the experiment), what is then the incentive for a true commitment and a best-response strategy?
References:
[1] Alpert, M., & Raiffa, H. (1969/1982). A progress report on the training of probability assessors.
[2] Bechara A., Damasio H., Damasio AR, Lee GP (1999) Different contributions of the human amygdala and ventromedial prefrontal cortex to decision-making. Journal of Neurosciences 19: 5473-5481.
[3] Bechara A., Damasio H., Damasio AR (2000) Emotion, Decision Making and the Orbitofrontal Cortex. Cerebral Cortex Mar, 10: 295-307.
[4] Wittmann, M. and Paulus, M.P. (2007), Decision making, impulsivity and time perception, Trends Cogn. Sci.
[5] Zajonc RB (1984) On the primacy of affect. American Psychology, 39: 117-123.